Intelligent Warehouse Optimization Using Real-Time Sensor Data and Machine Learning
Research:
As the lead contributor to an advanced supply chain optimization project at the University of North Texas, I directed a data-driven initiative to enhance warehouse logistics through the integration of smart technologies and machine learning. The research centered on improving forklift driver efficiency, minimizing idle time, and advancing safety protocols using smart glasses (Argus Science), iMotion sensors, and real-time behavioral tracking. I built and deployed machine learning models such as Random Forest and K-Means clustering, cleaned and analyzed large datasets with Python and SQL, and designed visual dashboards in Power BI to translate insights into strategic actions for operations teams.
​
​
Impact & Innovation:
This project played a pivotal role in modernizing ERP and MRP systems by embedding real-time data streams into inventory management, predictive maintenance, and resource planning. It introduced innovative methods like AR-guided forklift navigation, digital twin simulations for warehouse layout planning, and gamified safety training using eye-tracking feedback. The outcomes not only optimized material handling and reduced operational costs but also set the foundation for semi-autonomous vehicle implementation and sustainability-driven logistics strategies, significantly elevating warehouse intelligence and agility.
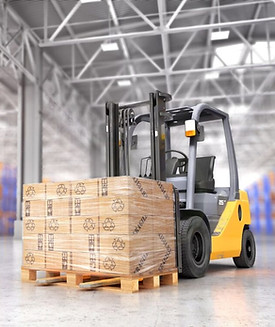